RESEARCH TRIANGLE PARK- RTI International data scientists, Rob Chew and Michael Wenger, have launched an open source project on GitHub called SMART: Smarter Manual Annotation for Resource-constrained collection of Training data. Breakthroughs in artificial intelligence resulting in human-level performance on complex tasks such as object and speech recognition are largely due to increases in computing power and the availability of open, labeled data sets than through recent algorithmic innovations. And while computational capacity has historically increased exponentially, gains in annotating data is still a time consuming, manual process. The SMART application is designed to help data scientists and research teams efficiently build labeled training datasets for supervised machine learning tasks. It aims to reduce manual coding time and effort, making machine learning classification tasks more affordable and widely accessible.
The SMART application is an annotation software that leverages elements of active learning and UI/UX design to reduce the effort of manual labeling training data. Active learning is a process in which learning algorithms actively query a user or other sources for data labels. SMART includes features such as active learning, inter-rater reliability, an administrator dashboard, multi-user coding, and on-premise install. SMART is open source under an MIT License.
Rob Chew was selected by the National Consortium for Data Science (NCDS) as one of two 2017-2018 NCDS Data Fellows to receive funding to support work that addresses data science research issues in novel and innovative ways. Part of the NCDS Data Fellows funding allowed Mr. Chew to mentor Michael Wenger in the shared development of the SMART application. Supplemental funding by RTI supported the development efforts of Caroline Kery, a summer intern in the RTI Center for Data Science, and efforts to open source the application.
Learn how RTI translates data into actional insights through Data Science.
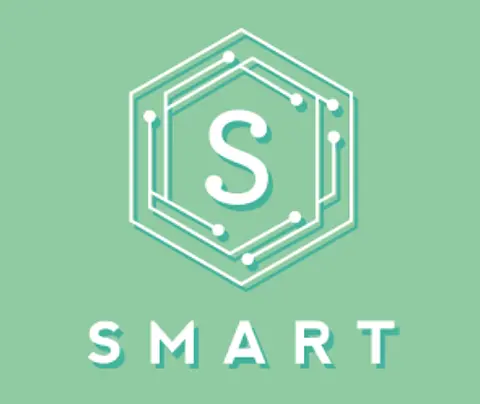
To request an interview, contact our Media Relations team.