Source: Photo by Sefik IIkin Serengil licensed under CC 4.0, Sefiks
In recent weeks, the Craiyon (formerly DALL-E mini) multimodal artificial intelligence (AI) model has soared in popularity on the internet. In this model, users input text prompts, which are paired with image inputs to generate newly created images. The images aim to depict text prompts and, as the model learns and responds to more requests, its self-generated images gradually match more closely with the requests of its users. As users have pushed to come up with increasingly bizarre and humorous image results, and these images have been widely reshared, the Craiyon has given the public a playful introduction to multimodal AI technology. However, the potential of multimodal AI deep learning goes far beyond creating shareable joke images—multimodal AIs have a wide range of use cases and will likely come to transform human lifeways and business organization.
Multimodal AI Technology's Influence – From Learning to Shopping & Beyond
One such example of how multimodal AI technology might heavily influence our lives is Facial Emotion Recognition (FER). FER AI models attempt to infer emotional states through analyzing facial expressions present in still images. Facial expressions are coded within the models to align with certain emotional states such as sadness, happiness, or anger. These models could be used to evaluate consumer behavior and help companies understand the popularity of certain products, packaging, or store designs by tracking changing emotional states of shoppers, or they might be used to evaluate learning in real time. By tracking changing emotional states of students, FER technology could help teachers better understand what teaching styles, lesson plans, and methodologies resonate most with their students.
These are just a couple of potential applications of this technology, but its utility is far broader. As multimodal AI technology becomes more refined, FER models will likely become far more ubiquitous in public life. However, FER technology has limitations. RTI’s Lab 58 is working to overcome these limitations by developing a multimodal, deep learning AI model, one that processes audio along with visual data.
What is Multimodal Learning?
Human beings process the world multimodally. We rely on a wide range of sensory inputs to arrive at conclusions or grasp concepts—audio, visual, text, tactile, etc. Humans are also frequently called to produce forms of feedback—text, for example—based on separate varieties of modal inputs, such as image or audio. For example, in our daily lives we might describe the content of images with speech or written text.
Similarly, AI models can be trained to produce outputs corresponding to data inputs of other modalities. Furthermore, they can be trained to produce outputs derived from multiple kinds of data inputs simultaneously. Importantly, output modalities of multimodal learning AI models tend to hold greater accuracy when multiple sources of modal inputs are present as the models learn. But importantly, human beings do not process information and arrive at conclusions with equal weighting given to the different modal inputs—they often privilege some modalities of data over others depending on situational contexts. AI models can also be designed and trained using weighted algorithms such that certain inputs count more heavily when producing outputs. And over time, as they are utilized more extensively, the processing abilities of multimodal deep learning AI models progressively advance, allowing them to produce more relevant, useful outputs.
Multimodal AI Shortcomings – Looks Can Be Deceiving
Much of the skepticism presently directed toward FER technologies concerns the accuracy of pairing facial expressions with certain emotional states. Although it is true that people do tend to produce certain facial expressions or movements when feeling certain emotions (e.g., smiling when happy) at a level significantly greater than chance, facial expressions and emotional states do not always correlate with one another. Modes of emotional expression and communication are not universal and vary significantly across different cultures as well as within specific situational contexts.
Contemporary FER may be unable to follow through on its promise of delivering concrete determinations of emotional states. This being said, human beings themselves are not infallible when interpreting others’ feelings, especially based on visual cues or facial expressions alone. Almost everyone can recall a situation in which they misread someone’s emotional state. As such, the present limitations of FER AI models do not necessarily represent a flaw with the technology itself but more so a difficulty produced by the non-universality of human social relations and emotional expression writ large. Just as human beings depend on further context clues and different sensory modes for detecting emotions (vocal tone and timbre, speech content, etc.), emotion recognition AI models might be improved by utilizing multimodal learning rather than relying solely on visual processing.
How Multimodal Learning Can Enhance Emotion Recognition Technology
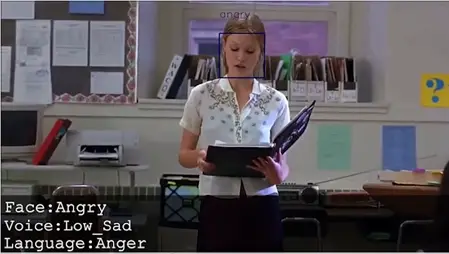
Figure 1. Screenshot of Lab 58 emotion recognition AI run over Julia Stiles' character in 10 Things I Hate About You. The AI model is detecting emotional signals and its interpretation is shown in the bottom left. Source: Lab 58 Computer Vision Technical Brief.
Lab 58 has developed a multimodal AI that infers emotional states based on FER, vocal tone, and content of language. An example of this model’s analysis is shown in Figure 1 above. By analyzing multiple modal inputs simultaneously, Lab 58’s AI model far more comprehensively infers emotional states than traditional FER models. By providing the ability to cross-reference detected emotional states through multiple modes of expression, Lab 58’s technology can draw far more informed outputs regarding emotional states.
Multimodal AI technology will be a massive gamechanger within the emotion recognition technology space, which will provide far more usable data for use cases such as evaluating student learning patterns or monitoring consumer retail behavior. With emotion recognition technology representing a burgeoning market space—$23.6 billion market in 2022 with a forecasted 12.9% compound annual growth rate through 2027—Lab 58, with its innovative multimodal technology, stands well-poised to advance the field of emotion recognition while helping companies incorporate transformative technology within their business models.