Coding offense texts: A need in the criminal justice research space
Criminal justice data frequently includes hundreds, if not thousands of free-text offense descriptions for a particular charge. Many criminal research projects require organizing this offense text into a reasonable number of meaningful categories to support analyses. One common approach is to group the charges by the National Corrections Reporting Program (NCRP) categorization.
Typically, an analyst would match the offense text with the relevant categorization using a lookup table. However, there are significant limitations to this approach with crime charge data. The following example illustrates these limitations with offense texts relating to the NCRP charge category “Auto Theft”:
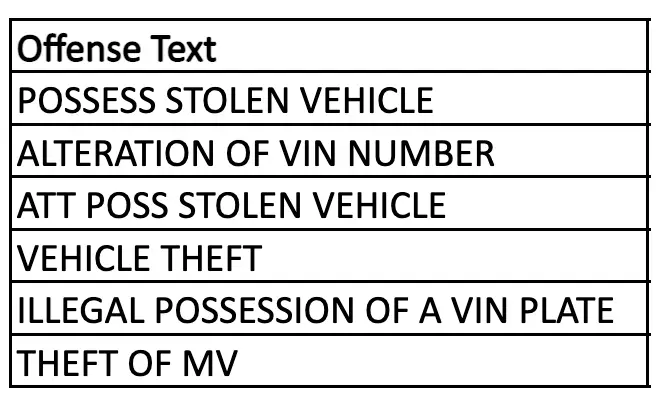
An analyst must be familiar with the abbreviations and acronyms in order to translate the offense text into something that is human-readable (e.g “poss” and “possess” are short for “possession”; “mv” stands for “motor vehicle”). Now that the offense text is understandable, a coder would need to know which NCRP charge category (e.g. “Auto Theft”) these offenses belong to, which requires an additional step of research.
Now imagine needing to be familiar with other common abbreviations, and even common misspellings, outside of the “Auto Theft” charge category. Additionally, there are cases where an abbreviation may have multiple meanings that may result in different charge categories applied to the same offense code within a research project.
Manually coding large datasets with a complex coding scheme can take days to weeks, depending on the number of records. Beyond the time involved, a manual process is subject to several sources of error: two analysts might code the same offense text differently or the same analyst might inconsistently apply codes. Adding extra time for quality control is a necessity. According to Debbie Dawes, Program Director of Court Systems Research at RTI, “Manually coding datasets is a major and common challenge on multiple criminal research projects.”
An easy-to-use coding tool that anyone can access
To meet this unique research need, RTI has developed the Rapid Offense Text Autocoder (ROTA) tool, an open-source machine learning model accessible in a web application allowing for the bulk upload and conversion of offense text into charge categories.
Let’s revisit our “Auto Theft” example above to see how the autocoder tool works in action.
1. The researcher goes to the ROTA web application and uploads their file to the Bulk Coder.
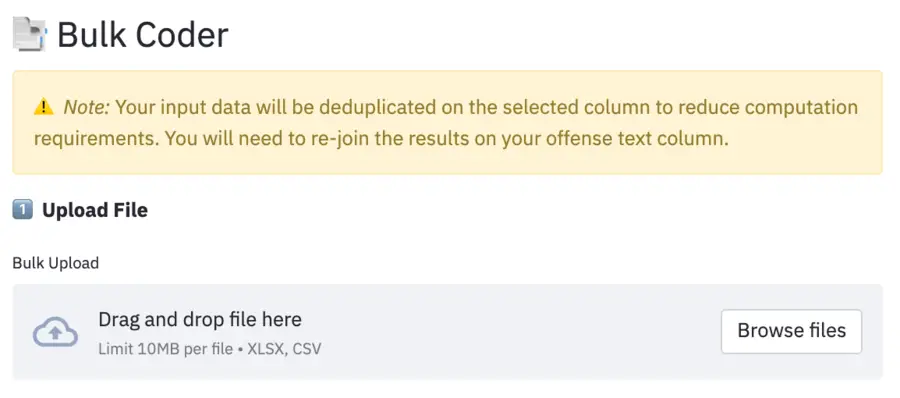
2. The web application automatically suggests a text column which may contain the offense text for coding into charge category.
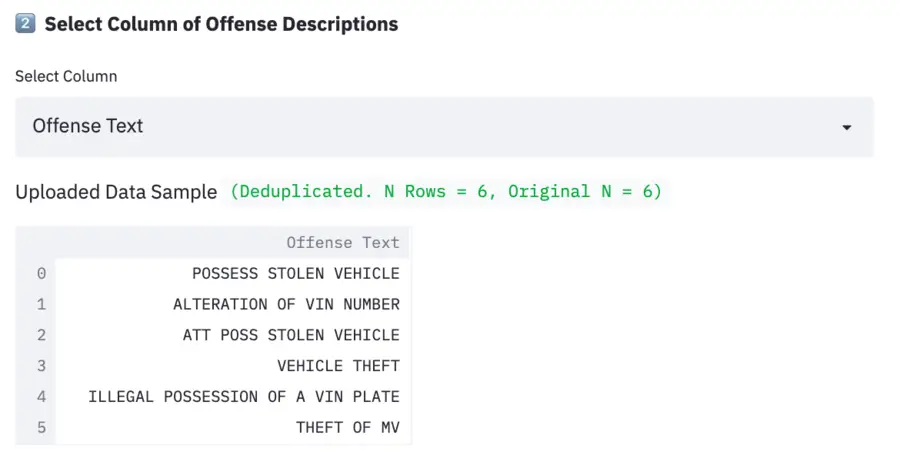
3. Once the correct column is selected, the researcher receives NCRP charge category predictions for each line in the offense text field and may download the CSV to further support their research.
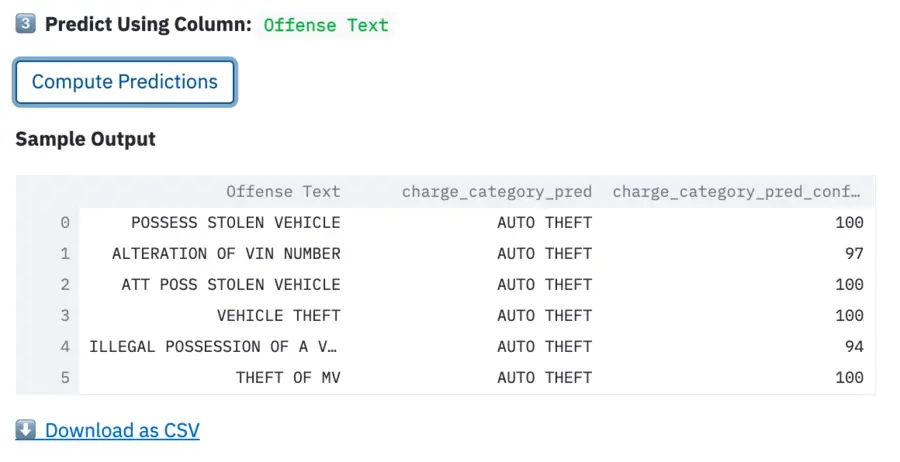
This process takes what was an arduous, days- or weeks-long data preparation task and returns predicted charge categories in a matter of seconds.
Behind the scenes: A fusion of machine learning and open-source at work
ROTA implements what is known in the Natural Language Processing (NLP) domain as a Transformer model. Transformer models provide a number of benefits over classic machine learning methods, chiefly high classification accuracy without substantial data present.
Transformer models derive much of their benefit from a concept called transfer learning, which is named such because these models can transfer general knowledge to our specific use case. For ROTA, this means that we’re able to take advantage of any general language information contained in a language model as well as any specific criminal justice text that was included within the original training data and build on it, rather than start that process from scratch.
The model used in ROTA is trained on a publicly available national lookup table combined with other hand-labeled offense text datasets. It yields an overall accuracy of 93% when predicting across 84 unique NCRP charge categories. The power of transfer learning is evident when evaluating performance on categories for which we have very little data – for example, the “Trafficking – Heroin” category has roughly 100 training observations, but on average ROTA is able to correctly classify 90% of the total number of examples in this category.
The model also outputs a confidence score for each assignment, which a researcher could use to identify which coded texts might need additional review, and which texts can be automatically coded with less concern. For example, if only predictions where the confidence score is above 95 are automated, this covers 93% of the data we used, and the model is 97% accurate on these texts.
For charge category-specific performance, see our open-source model repository for the latest trained model embedded in the web application and its performance statistics. RTI decided to open-source our model to provide transparency regarding the model performance and to enable researchers to continue model development for their similar data and/or tasks. For example, they can continue training the model or fine-tune model weights.
ROTA utilizes additional open-source technology in the form of the streamlit library and hosting services to bring the tool to a web interface. Streamlit enabled our developers to quickly and easily stand up a web application to make the model predictions accessible in a way that is just as quick and easy for researchers in the field to use.
Utilizing NCRP charge categories is only one method of training the ROTA tool to make predictions. Future iterations of ROTA may include additional categorization schemes for offense texts.